Unlocking Multilingual Capabilities with Azure AI's Cohere Embed V3 Model
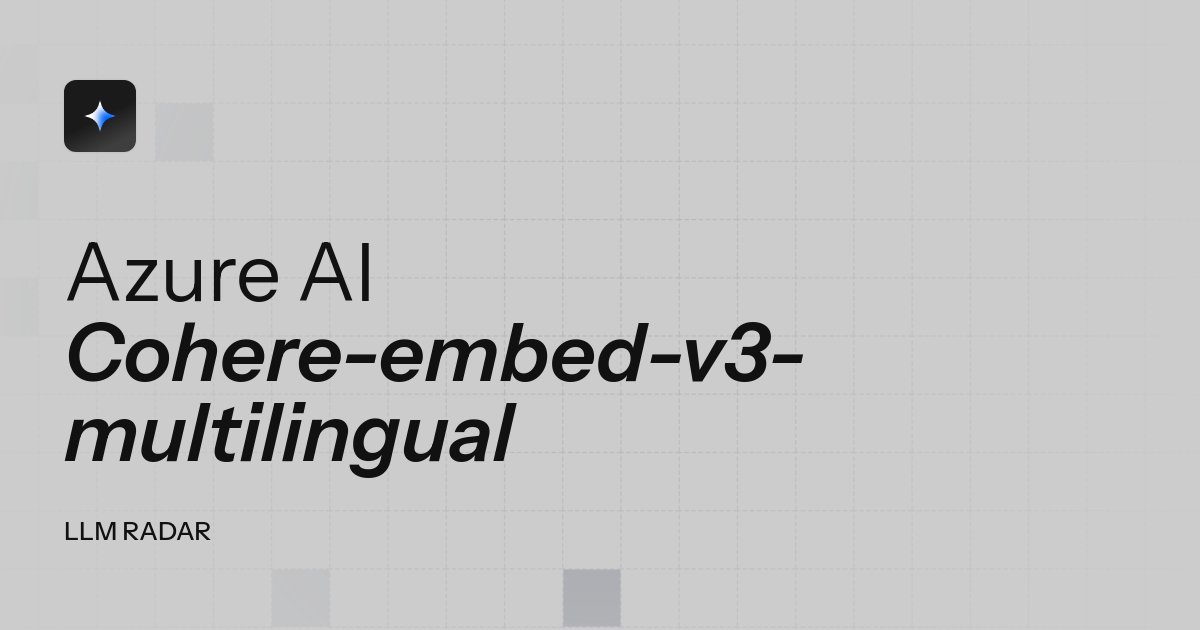
Azure AI has integrated the latest Cohere Embed V3 Multilingual model, a cutting-edge tool for advanced semantic search and text-based applications. Let's explore the key features, use cases, and deployment options that make this model a game-changer.
Key Features
- Multilingual Support: Supports over 100 languages for seamless cross-language searching and retrieval.
- Dimensions and Performance: 1024 dimensions with state-of-the-art performance on MTEB and BEIR benchmarks.
Use Cases
- Semantic Search: Optimized for evaluating and ranking document content quality.
- Retrieval-Augmented Generation (RAG): Enhances RAG systems for more insightful responses.
- Classification and Clustering: Performs well across various industries such as finance and legal.
Deployment and Integration with Azure AI
- Serverless API Endpoints: Deployable via Azure AI Studio, Azure Machine Learning SDK, Azure CLI, or ARM templates with pay-as-you-go billing.
- Azure AI Search: Integrates efficiently, supporting int8 embeddings for significant memory and speed improvements.
Technical Details
- Input Types: Generates document or query embeddings tailored to specific use cases.
- API Usage: Available through Cohere API or Azure AI's native API schema.
Efficiency and Cost
- Compression-Aware Training: Reduces the cost of running large vector databases without compromising search quality.
- Memory Optimization: Utilizes int8 embeddings for 4x memory savings and 30% speed-up in search.
Overall, the Cohere Embed V3 Multilingual model is an advanced tool that enhances semantic search, RAG, and other text-based applications, offering efficient integration with Azure AI services.