Exploring Cohere's Embed-English-V2.0: A Classic Embedding Model for English Texts
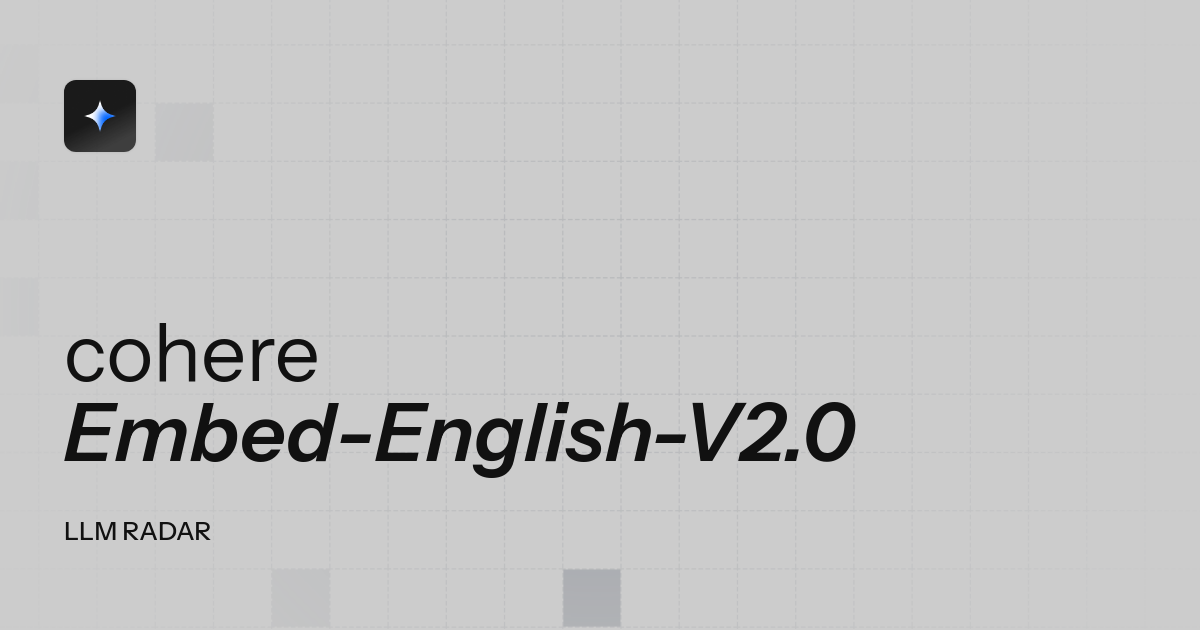
In the rapidly evolving landscape of language models, Cohere's Embed-English-V2.0 remains a relevant tool for specific text processing tasks. While not the latest in Cohere's lineup, this model serves as a reliable option for generating embeddings for English texts.
Model Overview
The Embed-English-V2.0 model is tailored for the English language, providing users with the capability to classify text or transform it into embeddings. It excels in generating embeddings with 4096 dimensions, allowing for a context length of up to 512 tokens. This specificity makes it suitable for focused applications where English text processing is required.
Key Features
- Embedding Dimensions: The model outputs embeddings in a high-dimensional space of 4096 dimensions, offering detailed textual representations.
- Similarity Metric: Cosine similarity is employed for comparing embeddings, ensuring effective semantic understanding.
- Endpoints: Users can access the model via the Classify and Embed endpoints, making it versatile for various applications.
- Embedding Type: Unlike the newer v3 models, this version supports only float embeddings, limiting certain advanced capabilities.
Applications
Despite its age, Embed-English-V2.0 is still useful for a range of tasks including text classification, semantic search, and clustering. These applications benefit from the model's ability to generate precise embeddings, although users seeking enhanced performance and additional features might consider Cohere's v3 models.
Conclusion
While Cohere's Embed-English-V2.0 might not boast the advanced features of its successors, it remains a valuable resource for projects focusing exclusively on English text embedding. For those applications where the latest technologies are not a necessity, this model offers a cost-effective and efficient solution.