Introducing Cohere Embed-Multilingual-v2.0: A State-of-the-Art Multilingual Embedding Model
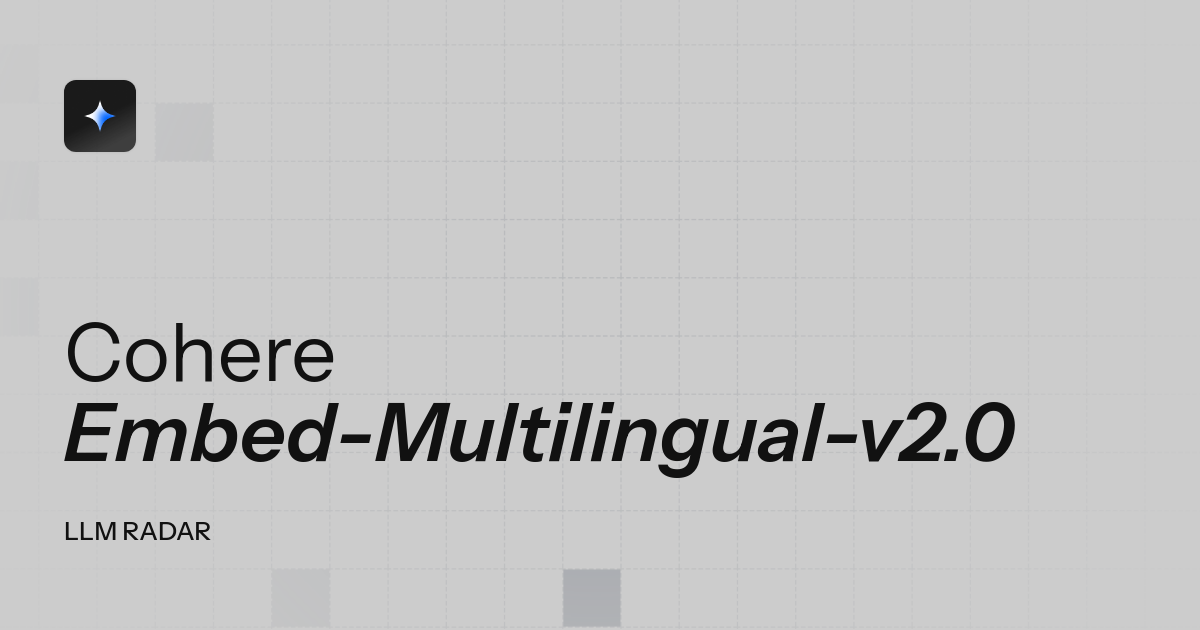
The Cohere Embed-Multilingual-v2.0 model represents a significant advancement in the field of natural language processing. This state-of-the-art multilingual embedding model is developed to support over 100 languages, making it an indispensable tool for applications that require language-agnostic text analysis and comparison.
Key Features
Multilingual Support
With support for over 100 languages, this model captures semantic meanings across different languages, enabling seamless text analysis and comparison irrespective of the language.
Embedding Dimensions
The embeddings generated by this model have 768 dimensions, allowing for a robust semantic representation of text, which is crucial for various NLP tasks.
Versatile Use Cases
Semantic Search
Cohere Embed-Multilingual-v2.0 facilitates semantic search by comparing the semantic similarity of text embeddings across different languages, making multilingual search more efficient and accurate.
Content Aggregation and Recommendation
This model can aggregate and recommend content in multiple languages, enhancing user experience by providing relevant information irrespective of language barriers.
Zero-Shot Cross-Lingual Text Classification
One of the standout features of this model is its ability to perform text classification across different languages without requiring explicit training data for each language.
Integration and Deployment
The model can be accessed through various platforms, including Amazon Bedrock, Amazon SageMaker, and other cloud services. It also supports deployment via SaaS API and will soon be available for private deployments (VPC and on-premise).
Performance and Scalability
Designed for high performance and scalability, the model supports data compression to reduce storage and compute requirements, ensuring efficient use of resources.
Technical Details
The model uses a dot product similarity metric and has a context length of 256 tokens. It can be integrated with other tools and frameworks, such as Langchain, to build complex text-based AI pipelines.
Applications and Examples
The model has been tested in various scenarios, including a multilingual QA system that can handle queries and provide responses in multiple languages seamlessly. It has also been used with vector databases like Weaviate to run complex NLP queries efficiently.
Overall, the Cohere Embed-Multilingual-v2.0 model is a powerful tool for bridging language gaps and enhancing the capabilities of NLP applications across diverse linguistic contexts.