Introducing Mixtral-8x7B: Mistral AI's Latest Breakthrough in Large Language Models
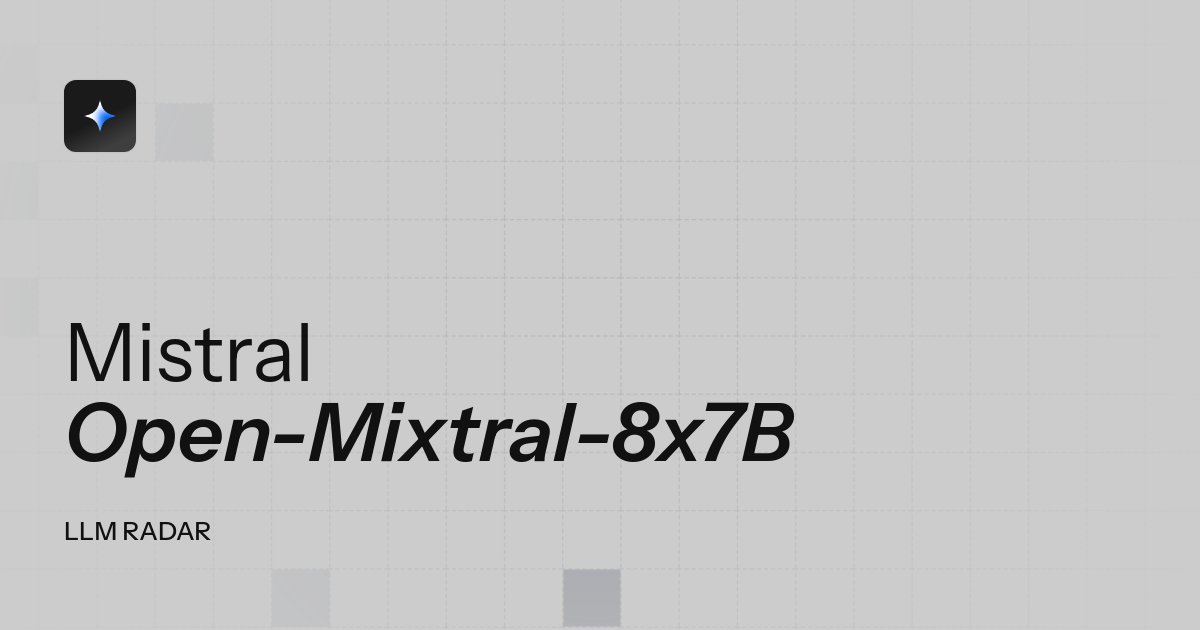
Mistral AI has unveiled its latest innovation in the realm of large language models (LLMs) with the release of Mixtral-8x7B. This state-of-the-art model offers a range of advanced features and capabilities designed to push the boundaries of AI performance and efficiency.
Architecture and Performance
At the core of Mixtral-8x7B lies the Sparse Mixture of Experts (SMoE) architecture. This innovative design allows the model to leverage up to 46.7 billion parameters while only utilizing about 12.9 billion parameters per token during inference. This results in enhanced inference throughput without a significant increase in computational cost.
In terms of benchmarks, Mixtral-8x7B outperforms Llama 2 70B on most tests and matches or exceeds GPT3.5 on standard benchmarks. It also boasts an impressive score of 8.3 on MT-Bench for instruction-following tasks.
Capabilities and Features
- Token Support: The model can handle a context of up to 32,000 tokens.
- Multilingual Support: Mixtral-8x7B is proficient in English, French, Italian, German, and Spanish.
- Code Generation: The model demonstrates strong performance in generating code.
- Inference Speed: It offers 6x faster inference compared to Llama 2 70B.
Licensing and Availability
Mixtral-8x7B is released with open weights under the Apache 2.0 license, making it accessible for community use and development. It is available via the Mistral AI API and can be deployed using an open-source stack, including integration with vLLM and Skypilot for cloud deployment.
Bias and Hallucination
The model presents less bias on the BBQ benchmark compared to Llama 2 and displays more positive sentiments on BOLD with similar variances.
Fine-Tuning and Instruction Following
An instructed version of Mixtral-8x7B, optimized for instruction following through supervised fine-tuning and direct preference optimization (DPO), is available. This version achieves a high score on MT-Bench.
Resource Requirements
While the model requires more vRAM due to its sparse architecture, it maintains efficient inference throughput.
Overall, Mixtral-8x7B represents a significant advancement in open-source LLMs. It offers a balance of performance, efficiency, and cost-effectiveness, making it a valuable tool for developers and AI enthusiasts.