Fine-Tuning Vertex AI's Code-Bison@002 for Enhanced Code Generation
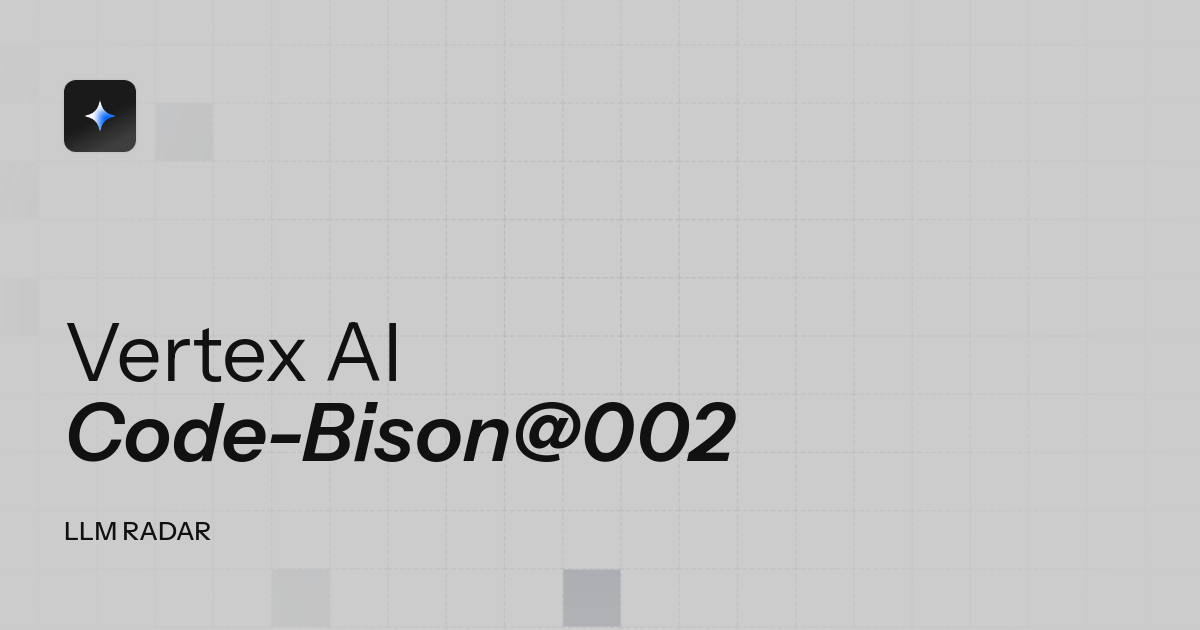
Vertex AI's latest offering, the code-bison@002
, is a powerful pre-trained model tailored for code generation tasks. Fine-tuning this model can significantly enhance its performance for your specific requirements. Here, we outline the streamlined process for fine-tuning and deploying this model using Vertex AI.
Steps to Fine-Tune a Pre-trained Code Generation Model
1. Load the Pre-trained Model
from vertexai.language_models import CodeGenerationModel
model = CodeGenerationModel.from_pretrained("code-bison@002")
2. Fine-Tune the Model
training_data = "gs://training/sample_data.jsonl"
model = model.tune_model(
training_data=training_data,
train_steps=100,
tuning_job_location="<LOCATION>",
tuned_model_location="<LOCATION>",
model_display_name="custom-code-gen-model",
accelerator_type="GPU"
)
3. Reuse the Fine-Tuned Model for Further Tuning
To fine-tune the model again, load the previously fine-tuned model from the Vertex AI model registry and repeat the tuning process. While Vertex AI does not automatically manage model versions, you can manually save models with distinct names or locations.
from vertexai.language_models import CodeGenerationModel
# Initial Fine-Tuning
training_data = "gs://training/sample_data.jsonl"
model = CodeGenerationModel.from_pretrained("code-bison@002")
model = model.tune_model(
training_data=training_data,
train_steps=100,
tuning_job_location="<LOCATION>",
tuned_model_location="<LOCATION>",
model_display_name="custom-code-gen-model",
accelerator_type="GPU"
)
# Load the Fine-Tuned Model for Further Tuning
fine_tuned_model = CodeGenerationModel.from_pretrained("custom-code-gen-model")
new_training_data = "gs://new_training/sample_data.jsonl"
fine_tuned_model = fine_tuned_model.tune_model(
training_data=new_training_data,
train_steps=100,
tuning_job_location="<LOCATION>",
tuned_model_location="<LOCATION>",
model_display_name="custom-code-gen-model-v2",
accelerator_type="GPU"
)
Key Points
- Model Loading: Use
CodeGenerationModel.from_pretrained
to load the pre-trained or previously fine-tuned model. - Fine-Tuning: Use the
tune_model
method to fine-tune the model with your custom dataset. - Model Management: Manage different versions of your fine-tuned models by saving them with different names or locations.
By following these steps, you can iteratively fine-tune your model with new data, consistently enhancing its code generation capabilities over time.