Introducing Voyage Rerank-2-Lite: A New Era in Latency-Optimized Reranking
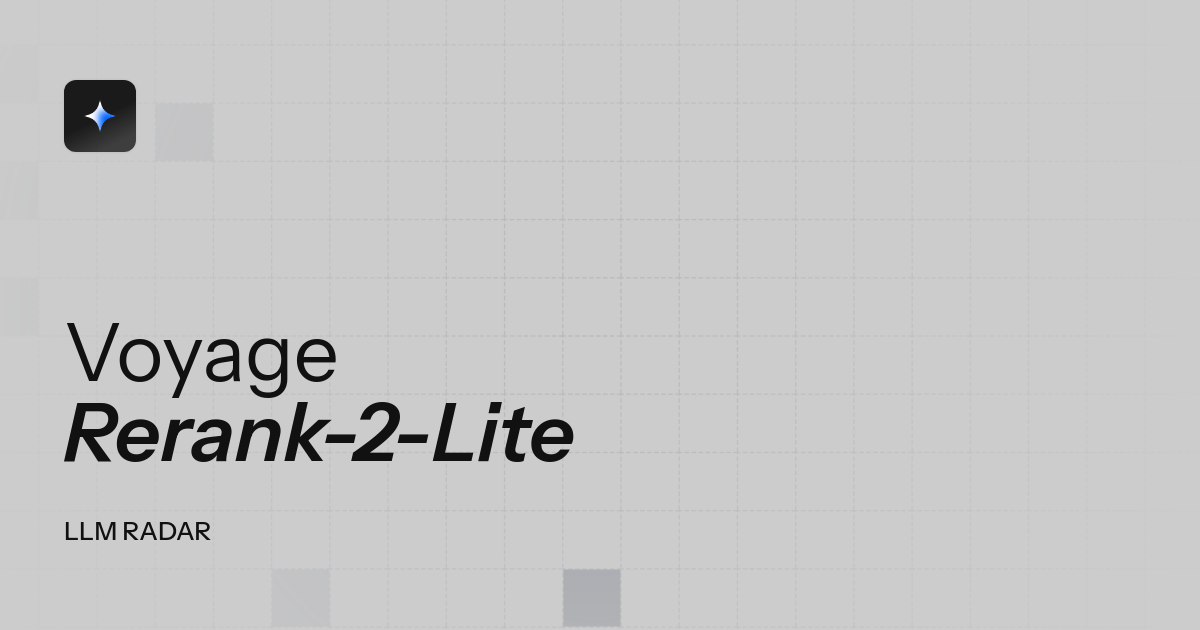
The world of AI-driven search is constantly evolving, and Voyage AI is leading the charge with the introduction of their latest reranker model, rerank-2-lite. This new model is designed with a focus on optimizing latency while delivering premium quality results. Here's why Voyage Rerank-2-Lite is set to redefine the standards in reranking technology.
Optimized for Latency
One of the standout features of rerank-2-lite is its optimization for latency. This model provides significantly lower latency, ensuring faster responses and a reduction in processing time. Despite the improvements in speed, rerank-2-lite maintains a high quality of output comparable to its predecessor, rerank-1, but with a 2.5x lower cost. This makes it an economically viable option without compromising on performance.
Enhanced Performance
When paired with OpenAI’s latest embedding model (v3 large), rerank-2-lite demonstrates impressive performance, improving accuracy by an average of 11.86%. It outperforms other models such as Cohere v3 and BGE v2-m3 by 5.12% and 13.59%, respectively. This boost in accuracy makes it an excellent choice for applications requiring precise and reliable search results.
Expanded Context Length
Rerank-2-lite supports a generous combined context length of 8K tokens for a query-document pair, which includes up to 2K tokens for the query. This is double the context length compared to similar models like the Cohere reranker, providing more room for comprehensive query-document interactions.
Multilingual Capabilities
This model is natively multilingual, outperforming Cohere multilingual v3 by 6.24% across 51 datasets in 31 languages. This feature ensures that rerank-2-lite can effectively handle a diverse range of languages, making it ideal for global applications.
Superior Domain-Specific Performance
In various domains, rerank-2-lite consistently outperforms other rerankers, including Cohere v3 and BGE v2-m3. This makes it a versatile tool for improving search scenarios across different fields and industries.
Easy Integration
Integration of rerank-2-lite into existing systems is straightforward. Users can simply specify the model in their API requests by using the Voyage API endpoint with the model set as rerank-2-lite. This ease of use allows quick upgrades and seamless transitions from previous models.
Recommendation for Users
Current users of rerank-lite-1 and rerank-1 are encouraged to upgrade to rerank-2-lite and rerank-2, respectively, to benefit from improved quality and increased context length, all at the same cost. This upgrade path offers a compelling opportunity for users to enhance their retrieval systems.
With its balance of performance, latency, and cost, rerank-2-lite is poised to be a game-changer in retrieval systems, offering a robust solution for a multitude of applications. Whether you're dealing with complex queries or diverse datasets, rerank-2-lite provides a reliable and efficient reranking solution.