Voyage-Code-3: Revolutionizing Code Retrieval with Next-Generation Embeddings
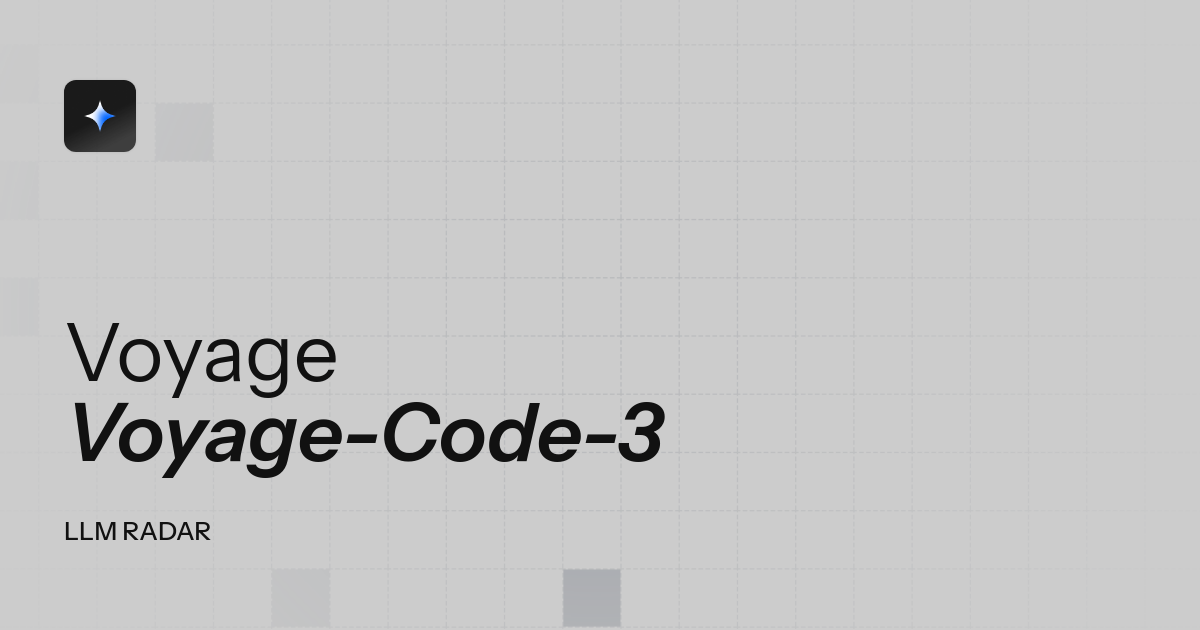
The landscape of code retrieval technology is rapidly evolving, and at the forefront of this revolution is Voyage-Code-3, the latest offering from Voyage AI. Engineered to redefine code retrieval efficiency, this next-generation embedding model is setting new benchmarks in the industry.
Unparalleled Performance and Efficiency
Voyage-Code-3 is designed to outperform existing models, boasting significant performance improvements over competitors such as OpenAI-v3-large and CodeSage-large. On average, Voyage-Code-3 achieves a remarkable 13.80% and 16.81% superiority across 32 code retrieval datasets. This leap in performance is not just limited to raw retrieval capabilities but extends to operational efficiencies as well.
One of the standout features of Voyage-Code-3 is its support for lower dimensional embeddings, ranging from 2048 to as low as 256 dimensions. This flexibility allows for reduced storage and search costs, crucial for large-scale applications, without sacrificing retrieval quality. Additionally, it supports various quantization formats, including float, int8, uint8, binary, and ubinary, further enhancing its adaptability and efficiency.
Advanced Learning and Training Techniques
The model incorporates innovative techniques such as Matryoshka learning and quantization-aware training. These methodologies enable Voyage-Code-3 to maintain high retrieval quality even at reduced dimensions and with quantized formats. For instance, at 1024 dimensions, it outperforms OpenAI-v3-large by 14.64%, and at 256 dimensions, the margin extends to 17.66%. The inclusion of binary rescoring can further amplify retrieval quality by up to 4.25%.
Extended Context and Integration
Another critical advantage of Voyage-Code-3 is its support for a 32K-token context length, which is significantly longer than the 8K offered by OpenAI and the 1K by CodeSage large. This extended context capability is pivotal for complex code retrieval tasks that require comprehensive context analysis.
Voyage-Code-3 is more than just an advanced retrieval model; it is an integral component of a broader initiative to enhance Retrieval Augmented Generation (RAG) systems. With backing from Databricks Ventures, Voyage AI is working towards integrating their models into the Mosaic AI Model Serving solution, promising to elevate the accuracy and efficiency of RAG applications.
In summary, Voyage-Code-3 represents a significant leap forward in code retrieval technology. Its combination of superior performance, cost efficiency, and integration capabilities positions it as a vital tool for developers and organizations aiming to optimize their code retrieval processes.